TCL Electronics has officially launched its 2025 range of Mini LED TVs in South Africa, introducing cutting-edge display technology, massive screen sizes, and cinematic…
Trends: What to expect from machine learning in 2023
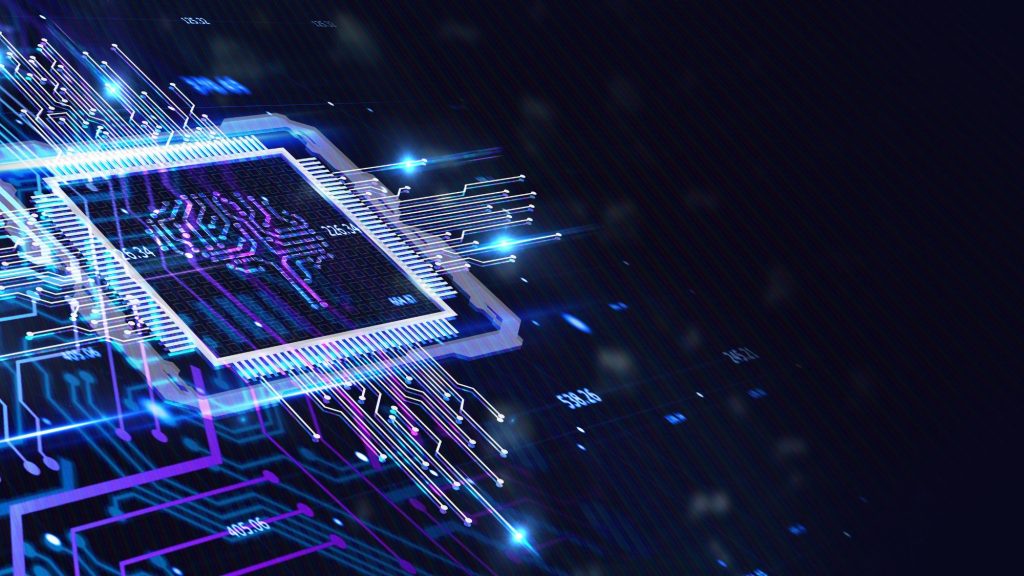
The leap Artificial Intelligence (AI) has made is expected to boost the digital transformation of businesses while disrupting various industries. Helm head of engineering Dr Colin Schwegmann, and natural language processing specialist Ari Ramkilowan provide trends to look out for in 2023.
This article was written by an actual Machine Learning model, along with Helm’s award-winning Natural Language Processing Specialist and their Head of Engineering. Trends to look out for in 2023.
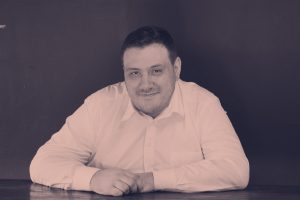
There aren’t many industries that are going to completely change over the next three years, but there are a few that are already making major shifts in how they operate.
Machine Learning holds significant promise for many of these high-growth industries, but it’s going to require significant changes in how we think about Machine Learning and data.
It is at the heart of nearly every industry in the world, from banking and insurance to retail and healthcare. With the recent rise in popularity of Artificial Intelligence and Machine Learning, it’s no wonder that more and more companies are investing in the technology.
Everything you’ve read until this point has been written by Helm’s Machine Learning model – yes, a bot – which was given only a headline and a single sentence to work with. This is similar to what the popular ChatGPT model has been doing for numerous users since the end of November 2022. Will we start to see more of this in the next year?
Globally, it’s predicted that in 2023 Artificial Intelligence (AI) will become more prevalent, along with Natural Language Processing and Machine Learning advancement – areas Helm has been innovating in for several years already. Along with computer vision, speech-to-text, and all marginal aspects of automation, we’ll see a massive impact on how technology is going to shape the future.
Helm head of engineering Dr Colin Schwegmann, and natural language processing specialist Ari Ramkilowan – a member of the team who recently won Wikimedia Foundation’s Research Award of the Year – weigh in on the three key trends they’re expecting to see and unpack how they will be adapted for the average person.
1. Unsupervised/self-supervised learning
Schwegmann explains there is a lot of data that is played with in different spaces e.g. images for image-based clients, and text for text-based clients. However, one of the most difficult parts is labelling that info, which entails adding specific human-verified names to images or text.
“There is so much data that cannot always be labelled. So we are starting to see an increase in self-supervised or unsupervised learning – using the data we have to learn the shapes and types of info we have instead of labelling it all – by finding patterns inside the data. We have successfully done this with Dr Oetker recently, where we’ve used high-res cameras and Computer Vision to create an AI-based application capable of identifying pizzas on the production line that do not meet the brand’s strict quality control standards.”
Self-supervised learning is where you provide unstructured data to a Machine Learning algorithm and ask it to learn patterns that become useful. A classic example of self-supervised learning for text is a language model.
Language models, such as GPT3, are fed with masses of text data and then trained to predict the next word based on the previous sentence, which is exactly what the model used in the first two paragraphs was trained to do. This also helps the AI to contextualise the data it’s given and reapply it for future tasks.
Self-supervised learning in imagery, however, is subtly different from text and is at the forefront of Machine Learning.
Ramkilowan says, “Step away from the paradigm of taking a model and showing it hundreds and hundreds of examples to distinguish between cats and dogs or overtopped and undertopped pizza. Or in text, is our user happy or unhappy at this point? In the past, we’d require hundreds or even thousands of examples to train a model.
“With prompt-based Zero-Shot Learning, you can now give your model a brief description of your task and it will give you the correct response without being explicitly trained to do so. We’re moving from a data-centric world (where mountains of data is needed by people with coding knowledge) to a people-centric one, where more and more people without any prior knowledge or training are able to leverage these technologies.”
“That’s the whole self-supervised approach to Machine Learning,” says Schwegmann, “it’s building our systems on the backs of giants.”
2. Natural language interfaces with technology
“Then moving from self-supervised learning, we’ll see an increase in both voice-only and multimodal-based applications. I predict more Natural Language Interfaces to be used with technology. For example, today Siri works mainly on an iPhone, sometimes on a laptop.
“In the future, these kinds of Natural Language Interfaces will be far more pervasive, available on more devices for more complicated tasks – think photo and video editing, but using your voice to instruct the machine,” explains Ramkilowan.
The ability for models to understand users’ intentions and execute tasks will improve dramatically over the next two to five years. “We are going to move – not away from the graphical user interface – but we are going to have a lot of natural language interfaces with our technology.
“So, communicating with self-driving cars is going to be a realistic future. The natural language interfaces will be a primary mode of communicating with these devices, and there is currently a focus on how to bridge this future with our own bots and other tools as we are still in the early stages.”
3. Decision intelligence
“We have lots of data that we use to train our systems, but on the flip side, we have lots of usage data that is not used. What could happen is that we see an increased efficiency in AI’s ability to discover hidden patterns in unstructured data, allowing us to unlock actionable insights and develop better guidelines and best practices at an inter and intra-organisational level.”
For any of these trends to transpire, there are limitations that will require adjustments and improvements but once these are made we will see these predictions unfold. For example, a trained text-based model doesn’t evolve over time and therefore won’t understand the changes that come about post-training e.g. the difference between the pre-Covid world and today.
What effect will this have on the average person?
One of the applications, Github Co-Pilot – which in one of its uses can be thought of as an auto-complete engine for software development – is built on OpenAI Codex, a model trained on billions of lines of code. Github Co-Pilot is capable of generating usable code to assist software developers to be more efficient and productive.
“Things like Github Co-Pilot and GPT3 are just scratching the surface of what’s possible. In the next wave of model breakthroughs we’ll likely see domain-specific proliferation in both creative and scientific fields (like Github Co-Pilot for video editing or biomedicine). We’ll be able to see these assisting creatives and helping scientists iterate and discover new things in equal measure,” explains Ramkilowan.
“Having the AI will help many fields become more efficient and more productive and accelerate progress in general at a rapid rate and we’ve seen that now with Github Co-Pilot.”
Five years ago, or even less, we would’ve said AI would be good at replicating menial tasks, or even those that require some skill. But what the industry has seen now is that it has been flipped on its head with the debate of even artistic areas veins affected by AI, e.g. TikTok filters.
It’s not about replacing jobs, it’s about making work or products better, and also improving efficiencies, sparking new ideas, and scaling. It is effectively putting the tech of Machine Learning into the hands of the average person, allowing them to pursue other interests with the time they would otherwise have spent on tasks AI now does.
Schwegmann concludes, “This way, people have more of an influence in how AI or Machine Learning is used going forward.”
For more information on Helm and the services it offers please visit www.helm.africa.
READ NEXT: Taking responsibility for using AI for good in Africa